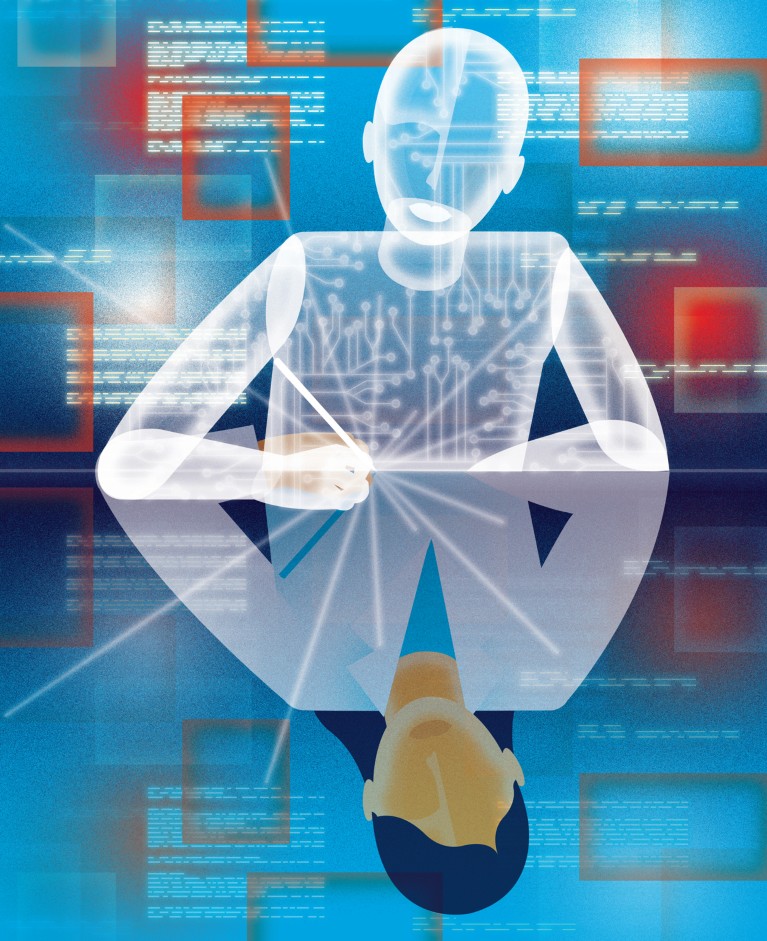
Illustration: Neil Webb
Do squirrel surgeons generate extra quotation affect? The query appears ludicrous, or maybe the beginning of a foul joke. However the query, posed by knowledge scientist, Mike Thelwall, was not a joke. It was a check. Thelwall, who works on the College of Sheffield, UK, had been assessing the flexibility of enormous language fashions (LLMs) to consider tutorial papers in opposition to the factors of the analysis excellence framework (REF), the UK’s nationwide audit of analysis high quality. After giving a customized model of ChatGPT the REF’s standards, he fed 51 of his personal analysis works into the mannequin and was stunned by the chatbot’s functionality to provide believable experiences. “There’s nothing within the experiences themselves to say that it’s not written by a human professional,” he says. “That’s an astonishing achievement.”
Nevertheless, the squirrel paper actually threw the mannequin. Thelwall had created the paper by taking one among his personal rejected manuscripts on whether or not male surgeons generate extra quotation impacts than feminine surgeons, and to make it nonsensical he changed ‘male’ with ‘squirrel’, ‘feminine’ with ‘human’ and any references to gender he switched to ‘species’ all through the paper. His ChatGPT mannequin couldn’t decide that ‘squirrel surgeons’ weren’t an actual factor throughout analysis and the chatbot scored the paper extremely.
Nature Index 2024 Synthetic intelligence
Thelwall additionally discovered that the mannequin was not notably profitable at making use of a rating based mostly on REF pointers to the 51 papers that have been assessed. He concluded that as a lot because the mannequin might produce authentic-sounding experiences, it wasn’t able to evaluating high quality.
The speedy rise of generative synthetic intelligence (AI) resembling ChatGPT and picture mills resembling DALL-E has led to rising dialogue about the place AI may match into analysis analysis. Thelwall’s research1, revealed in Might, is only one piece of a puzzle that teachers, analysis establishments and funders try to piece collectively. It comes as researchers additionally grapple with the various different ways in which AI is affecting science and the creating pointers which can be arising round its use. These discussions, nevertheless, have not often targeted on offering a steer on how AI may be utilized in assessing analysis high quality. “That’s the subsequent frontier,” says Gitanjali Yadav, a structural biologist at India’s Nationwide Institute of Plant Genome Analysis in New Delhi, and member of the AI working group on the Coalition for Advancing Analysis Evaluation, a worldwide initiative to enhance analysis evaluation observe.
Notably, the AI growth additionally coincides with rising calls to rethink how analysis outputs are evaluated. Over the previous decade, there have been calls to maneuver away from publication-based metrics resembling journal affect components and quotation counts, which have proven to be vulnerable to manipulation and bias. Integrating AI into this course of at such a time gives a possibility to include it in new mechanisms for understanding, and measuring, the standard and affect of analysis. Nevertheless it additionally raises vital questions on whether or not AI can absolutely support analysis analysis, or whether or not it has the potential to exacerbate points and even create additional issues.
High quality assessments
Analysis high quality is tough to outline, though there’s a normal consensus that good high quality analysis is underpinned by honesty, rigour, originality and affect. There’s all kinds of mechanisms, every working at totally different ranges of the analysis ecosystem, to evaluate these traits, and myriad methods to take action. The majority of research-quality evaluation occurs within the peer-review course of, which is, in lots of circumstances, the primary exterior high quality evaluate carried out on a brand new piece of science. Many journals have been utilizing a suite of AI instruments to complement this course of for a while. There’s AI to match manuscripts with appropriate reviewers, algorithms that detect plagiarism and verify for statistical flaws, and different instruments aimed toward strengthening integrity by catching knowledge manipulation.
Extra not too long ago, the rise of generative AI has seen a rush of analysis aimed toward exploring how nicely an LLM may be capable of support peer evaluate — and whether or not scientists would belief these instruments to take action. Some publishers permit AI to help in manuscript preparation, if adequately disclosed, however don’t permit its use in peer evaluate. Even so, there’s a rising perception amongst teachers within the capacity of those instruments, notably these based mostly on pure language processing and LLMs.
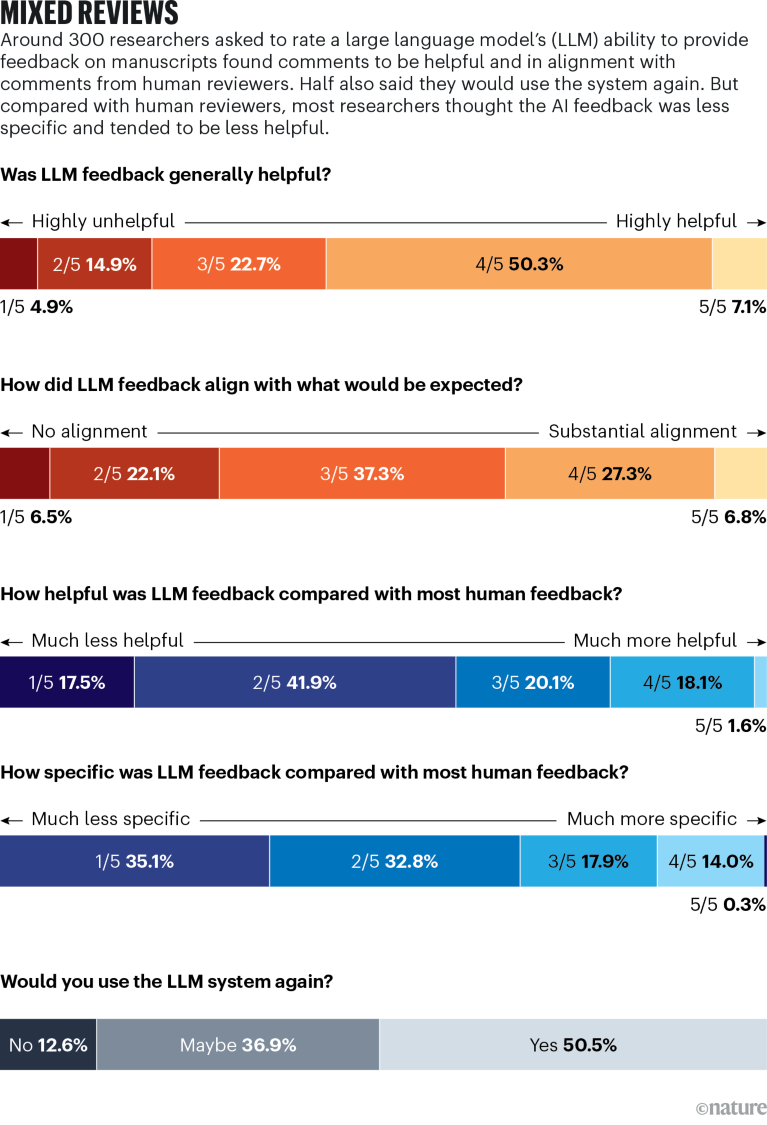
Supply: Ref. 2
A research revealed in July this yr2, led by pc science PhD scholar, Weixin Liang, within the lab of biomedical knowledge scientist, James Zou, at Stanford College in California, assessed the potential of 1 LLM, GPT-4, to supply suggestions on manuscripts. The research requested researchers to add a manuscript and have it assessed by their AI mannequin. Researchers then accomplished a survey evaluating the suggestions and the way it in contrast with human reviewers. It obtained 308 responses, with greater than half describing the AI-generated opinions as “useful” or “very useful”. However the research did spotlight some issues with that suggestions: it was typically generic and struggled to supply in-depth critiques.
Zou thinks this doesn’t essentially preclude the usage of such instruments in sure conditions. One explicit instance he mentions is early-career researchers engaged on the primary draft of a paper. They might add a draft to a bespoke LLM and obtain commentary about deficiencies or errors of their draft. However given the laborious and considerably repetitive nature of peer evaluate, some teachers fear that there may very well be an inclination to lean on the outputs from a generative AI system able to delivering experiences. “There’s no type of glory or funding related to peer evaluate. It’s simply seen as a scientific obligation,” says Elizabeth Gadd, head of analysis tradition and evaluation at Loughborough College, UK. There may be already proof that peer reviewers are utilizing ChatGPT and different chatbots to some extent, regardless of the foundations put in place by some journal publishers.
Thelwall believes there’s extra that AI might do in serving to peer reviewers to guage analysis high quality, however there’s motive to maneuver slowly. “We simply want numerous testing,” he says. “And never simply technical testing, but in addition pragmatic testing, the place we acquire confidence that if we offer the AI to the reviewers, for instance, that they received’t abuse it.”
Yadav sees nice profit in AI as a time-saving software and has been working with it to assist quickly assess wildlife imagery from field-based cameras in India, however she sees peer evaluate as too vital to the scientific neighborhood handy over to the bots. “I’m personally completely in opposition to peer evaluate being executed by AI,” she says.
High quality financial savings
One of the crucial mentioned advantages of utilizing AI is the concept that it might release time. That is notably obvious in institutional and nationwide techniques of evaluating analysis — a few of which have integrated AI. As an illustration, one funder in Australia, the Nationwide Well being and Medical Analysis Council (NHMRC), already makes use of AI by means of “a hybrid mannequin combining machine studying and mathematical optimisation methods” to determine appropriate human peer reviewers to evaluate grant proposals. The system helps to take away one of many administrative bottlenecks within the analysis course of, nevertheless it’s the place the AI use ends. An NHMRC spokesperson says the company “doesn’t use synthetic intelligence, in any type, to instantly help with analysis high quality analysis” itself.
Even utilizing AI for such administrative assist may very well be a significant useful resource saving, nevertheless, particularly for big nationwide assessments such because the REF. Thelwall says the train is understood for its unimaginable drain on researchers’ time. Greater than 1,000 teachers assist to evaluate analysis high quality within the REF and it takes them about half a yr to get it executed.
“If we are able to automate evaluations”, says Thelwall, then “it might be a large productiveness increase”. And there’s potential for big financial savings: the latest REF, in 2021, was estimated to have price round £471 million (US$618 million).
Equally, New Zealand’s evaluation of researchers, the Efficiency Primarily based Analysis Fund, has beforehand been described by Tim Fowler, chief government of the federal government’s Tertiary Schooling Fee, as a “backbreaking” train. In it, teachers submit portfolios for evaluation, inserting an excessive burden on them and establishments. In April, the federal government scrapped it and a working group has been charged with delivering a brand new plan by February 2025.
These examples counsel AI’s main potential to create extra effectivity, no less than for big, bureaucratic, evaluation techniques and processes. On the identical time, the expertise is creating as views on what constitutes analysis high quality are evolving and changing into extra nuanced. “The way you might need outlined analysis high quality within the early twentieth century isn’t the way you outline it now,” says Marnie Hughes-Warrington, deputy vice-chancellor of analysis and enterprise on the College of South Australia in Adelaide. Hughes-Warrington is a member of the Excellence in Analysis Australia transition group, which is contemplating the way forward for the nation’s evaluation train after a evaluate in 2021 discovered that it positioned a major burden on universities. She says the analysis neighborhood is more and more recognizing the necessity to evaluate extra “non-traditional analysis outputs” — resembling coverage paperwork, artistic works, exhibitions — after which past to social and financial impacts.
Because the conversations are occurring alongside the AI growth, it is smart that new instruments might match into revised strategies of research-quality analysis. As an illustration, Hughes-Warrington factors to how AI is already getting used to detect picture manipulation in journals or to synthesize knowledge from techniques used to uniquely determine researchers and paperwork. Making use of these sorts of strategies could be in line with the mission of establishments resembling universities and nationwide our bodies. “Why wouldn’t organizations, pushed by curiosity and analysis, implement new methods of doing issues?” she says.
Nevertheless, Hughes-Warrington additionally highlights the place incorporating AI will meet resistance. There’s privateness, copyright and data-security issues to acknowledge, inherent biases within the instruments to beat and a necessity to contemplate the context through which analysis assessments happen, resembling how impacts will differ throughout disciplines, establishments and nations.
Gadd isn’t in opposition to incorporating AI and says she is noticing it seem extra usually in discussions round analysis high quality. However she warns that researchers are already one of the crucial assessed professions on this planet. “My very own normal view on that is that we assess an excessive amount of,” she stated. “Are we utilizing AI to unravel an issue that’s of our personal making?”
Having seen how bibliometrics-based assessments can harm the sector, with metrics resembling journal affect components misused as an alternative choice to high quality and proven to hinder early-career researchers and variety, Gadd is worried about how AI may be applied, particularly if fashions are skilled on these identical metrics. She additionally says selections involving allocation of promotions, funding or different rewards will at all times want human involvement to a far higher extent. “It’s a must to be very cautious”, she says, about shifting to expertise “to make selections that are going to have an effect on lives”.
Gadd has labored extensively in creating SCOPE, a framework for accountable analysis analysis by the Worldwide Community of Analysis Administration Societies, a worldwide group that brings analysis administration societies collectively to coordinate actions and share data within the area. She says one of many key rules of the scheme is to “consider solely the place crucial” and, in that maybe, there’s a lesson for the way we must always take into consideration incorporating AI. “If we evaluated much less, we might do it to a better customary,” she says. “Perhaps” AI can assist that course of, however a “lot of the arguments and worries we’re having about AI, we had about bibliometrics.”