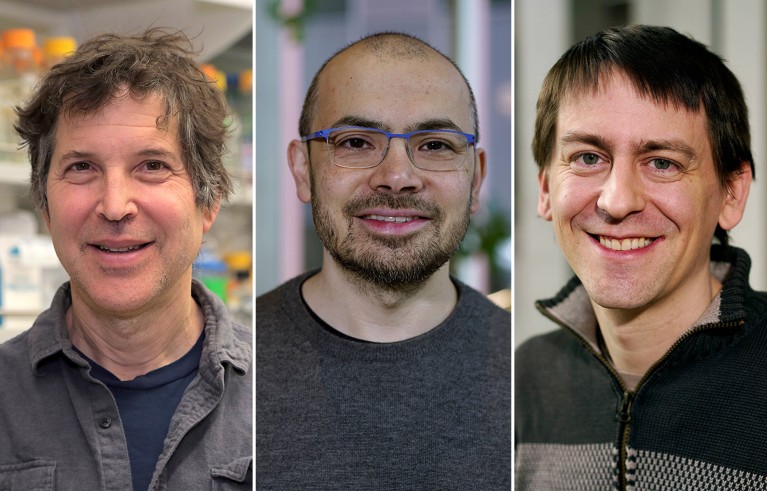
David Baker, Demis Hassabis and John Jumper (left to proper) received the chemistry Nobel for growing computational instruments that may predict and design protein buildings.Credit score: BBVA Basis
For the primary time — and doubtless not the final — a scientific breakthrough enabled by synthetic intelligence (AI) has been acknowledged with a Nobel prize. The 2024 chemistry Nobel was awarded to John Jumper and Demis Hassabis at Google DeepMind in London, for growing a game-changing AI instrument for predicting protein buildings referred to as AlphaFold, and David Baker, on the College of Washington in Seattle, for his work on computational protein design, which has been bolstered by Al previously few years.
“I hope once we look again on AlphaFold, it will likely be the primary proof level of AI’s unbelievable potential to speed up scientific discovery,” Hassabis stated at a press briefing at DeepMind on 9 October. “It’s so unreal at this second.”
What’s subsequent for AlphaFold and the AI protein-folding revolution
The impression of AlphaFold, which was unveiled just some years in the past, has been nothing wanting transformative. The instrument has made protein buildings — typically, however not all the time, extremely correct ones — accessible to researchers on the contact of a button, and enabled experiments that had been unimaginable a decade in the past. “It’s a significant revolution,” says Christine Orengo, a computational biologist at College Faculty London, whose laboratory has used AlphaFold-predicted buildings to uncover new proteins.
“It has lengthy been a dream to be taught to foretell the three-dimensional construction of proteins from understanding their amino acid sequences. For a number of a long time, this was thought-about inconceivable,” stated Nobel committee chair Heiner Linke, who researches nanoscience at Lund College in Sweden, in the course of the prize announcement. This 12 months’s laureates “have cracked the code”, he added. The three winners share a prize pot of 11 million Swedish kronor (US$1 million).
Award-winning AI
DeepMind debuted AlphaFold in 2018, when it received a biennial protein-structure prediction contest referred to as the Vital Evaluation of Protein Construction Prediction (CASP). Nevertheless it was the second iteration of the deep-learning neural community1,2, revealed in late 2020, that basically shook up the life sciences. Lots of AlphaFold2’s predictions at CASP had been so correct as to be indistinguishable from experimentally solved protein buildings.
Hassabis, DeepMind’s co-founder and chief govt, and Jumper, head of the AlphaFold crew, led the event of AlphaFold2. To foretell protein buildings, the neural community incorporates information from libraries of a whole bunch of 1000’s of buildings and thousands and thousands of sequences from associated proteins — which maintain details about their shapes.
win a Nobel prize: what sort of scientist scoops medals?
Specifically, AlphaFold’s success is due in no small half to the Protein Information Financial institution, a freely accessible repository of greater than 200,000 protein buildings decided utilizing strategies together with X-ray crystallography and cryo-electron microscopy. “It’s humbling each time we prepare [AlphaFold] on years of effort. Every information level is years of effort from somebody,” Jumper stated on the DeepMind press briefing.
In 2021, DeepMind made AlphaFold2’s underlying code freely accessible, together with the info wanted to coach the mannequin. An AlphaFold database, created with the European Molecular Biology Laboratory’s European Bioinformatics Institute in Hinxton, UK, now holds the buildings of just about all of the proteins from each organism represented in genetic databases, some 214 million predictions in complete. This 12 months, the corporate unveiled a third model of AlphaFold, which might mannequin different molecules that work together with proteins, similar to medicine3.
The revolution that Jumper, Hassabis and their colleagues unleashed remains to be in its early days, and AlphaFold’s full impression on science may not be recognized for years. Already, the instrument helps scientists make new insights.
One pioneering crew used the instrument, together with experimental information, to map the nuclear pore advanced, certainly one of our cells’ largest machines that transports molecules into and out of the nucleus4. Final 12 months, two groups mined your complete AlphaFold database to uncover the darkest corners of the protein universe, figuring out new households of proteins and folds and shocking connections within the equipment of life5.
‘The whole protein universe’: AI predicts form of practically each recognized protein
Many researchers hope that AlphaFold and different AI instruments it has impressed will rework drugs, however it’s not but clear how, or certainly whether or not, AlphaFold will streamline the pricey and multi-step technique of growing secure medicine. Scientists laying the groundwork for brand new vaccines are discovering AlphaFold extremely helpful and, in some instances, recreation altering. However AlphaFold is a complement to experimental research and different approaches to mapping and tweaking the construction of viral proteins to be used in vaccines.
For many researchers, a predicted construction is the start of a examine, not the tip, says Jan Kosinski, a structural modeller on the European Molecular Biology Laboratory in Hamburg, Germany. “Originally, there was this worry that it’s going to change structural biology, individuals will lose jobs and so forth. Truly, the exact opposite has occurred,” he provides.
David Jones, a bioinformatician at College Faculty London who collaborated with DeepMind on the primary model of AlphaFold beginning in 2016, says one of many instrument’s largest impacts has been a change in biologists’ mindsets, individuals realized “that computer systems are issues that may produce helpful hypotheses that may be examined within the lab”.
Creating new proteins
Some 20 years earlier than DeepMind began engaged on AlphaFold, computational biophysicist David Baker and his colleagues developed a software program instrument referred to as Rosetta that modelled protein buildings utilizing bodily rules. The instrument compares small fragments of a number of present protein buildings and sequences to determine a protein sequence that may fold into a specific form.
Initially, Rosetta was utilized to predicting protein buildings — it has been among the many high entries at quite a few CASPs, earlier than AlphaFold’s dominance. However Baker quickly realized that the mannequin might be flipped round to design solely new proteins.
AI instruments are designing solely new proteins that would rework drugs
The instrument had early success in designing proteins6, together with new sorts of enzyme, proteins that may bind tightly to different molecules and self-assembling protein nanoparticles that resemble viruses (certainly one of these served as a foundation for an authorised COVID-19 vaccine).
When AlphaFold2 was introduced — however not but launched — Baker and his crew, together with computational chemist Minkyung Baek, now at Seoul Nationwide College, got down to perceive the software program and apply a few of its methods to a earlier AI-based model of Rosetta. The primary model of the ensuing RoseTTAFold community7 carried out practically in addition to AlphaFold2. Since 2021, each networks have been frequently improved by their builders and different scientists to sort out recent challenges, similar to predicting the construction of complexes of a number of distinct interacting proteins.
Baker’s crew has been particularly prolific in making use of machine studying to his lab’s raison d’etre: creating new proteins by no means earlier than seen in nature. A instrument lately developed by Baker’s crew that mixes RoseTTAFold with image-generating diffusion neural networks has led to a step change in researchers’ capability to design proteins.
Speedy progress
Such instruments have been a large accelerator and democratizer, says Sergey Ovchinnikov, an evolutionary biologist on the Massachusetts Institute of Expertise in Cambridge, who did his PhD in Baker’s lab. It used to take Rosetta weeks of operating on a whole bunch of processors to provide you with a protein design, a job that newer AI-based instruments can accomplish in seconds. “Now everyone on the earth can do protein design,” he says.
“I’ve been actually deeply impressed by the others within the discipline and the individuals I’ve labored with,” stated Baker, talking by phone on the Nobel prize announcement. “I stood on the shoulders of giants.”
Medication Nobel awarded for gene-regulating ‘microRNAs’
Martin Steinegger, a computational biologist at Seoul Nationwide College, likens the impression of AlphaFold, RoseTTAFold and different organic AI instruments to that of the Apollo Moon missions, as a result of they present what engineering can obtain. “It is a related second for construction prediction and the structural biology discipline — simply seeing what is feasible,” he says.
Few had been stunned with the Nobel committee’s resolution. For Baker, “most individuals thought that it was ‘a when not if’ state of affairs, the quantity of labor he’s finished in that discipline,” says Jones. Jumper, conscious that he and Hassabis had been on many individuals’s shortlists, stated within the press briefing that he was unable to sleep the evening earlier than at present’s announcement.
For Jumper, the anticipated buildings that AlphaFold delivers create alternatives for scientific discovery. Tens of millions of scientists have already used the instruments, and he hopes that it received’t be lengthy earlier than certainly one of them will get a name from Sweden. “The second that I shall be virtually as excited as this would be the Nobel prize that talks concerning the work finished with AlphaFold,” he stated.